Categories
AI in education
21 January 2025How do we ensure our AI-generated resources are high-quality?
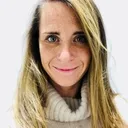
Hannah-Beth Clark
AI Quality and Safety Principal
We're taking the need for accuracy, safety and pedagogical rigour really seriously with Aila, our AI lesson assistant. Today our paper, Auto-Evaluation: A Critical Measure in Driving Improvements in Quality and Safety of AI-Generated Lesson Resources, has been published by MIT Open Learning. This milestone highlights our work to ensure AI-generated lesson content not only reduces teacher workload but also drives the highest quality standards.
What did we do?
Aila, our AI lesson assistant, can support teachers with creating lessons efficiently and reducing workload - but how do we ensure the quality of the lessons? To address this, we developed an auto-evaluation tool (a tool that uses AI to judge the quality of AI-generated content) capable of quickly evaluating lessons across multiple subjects and key stages. Over the course of the study, we evaluated 4,985 AI-generated lessons in English, Maths, Science, Geography, and History.
How we measured quality
We used our AI-powered auto-evaluation tool to evaluate lessons against a set of 24 quality benchmarks, with a key focus for this paper on multiple-choice questions and distractors (wrong answers in multiple-choice questions). Experienced teachers also reviewed a selection of these multiple choice questions, so that we could compare their score and justification against the auto-evaluation tool’s score and justification.
By analysing patterns in low- and high-quality distractors, we identified some key themes in the AI-generated quizzes:
- Low-quality distractors: included opposite sentiments to correct answers, mismatched grammar, or obvious choices that repeated question words.
- High-quality distractors: incorporated common misconceptions, shared thematic consistency, and featured similar grammatical structures to correct answers.
Driving improvements
Armed with these insights, we codified our findings with accompanying examples and incorporated this into our auto-evaluation tool’s prompt (the instructions we have given to the tool). The results were significant:
- Our AI powered auto-evaluation tool made judgements more aligned with expert human teachers. We know this because the difference between auto- and human evaluations decreased (mean squared error reduced from 3.83 to 2.95) and the agreement between these evaluations increased (from 0.17 to 0.32 measured using a Quadratic Weighted Kappa).
- We were able to incorporate these findings into Aila’s prompt, driving the quality of content produced.
These results give us clear, actionable insights to guide improvements in the lessons Aila produces.
This work also showcases the opportunity to use AI-powered evaluation tools to evaluate the quality of AI content. It highlights the importance of using human evaluation to corroborate and improve the alignment of these auto-evaluation tools to the judgments of expert humans.
What we learned
- Start with quality: Access to high-quality underlying materials (such as our open-source corpus of lessons and resources) is essential for building effective AI tools.
- Codify excellence: Defining and exemplifying excellence within your organisation helps to guide AI tools in producing high quality content and enables you to set clear benchmarks for evaluation.
- Iterative evaluation: Cycles of auto- and human evaluation refine tools over time, driving up quality and consistency.
Find out more
To read our full findings, check out our paper published by MIT Open Learning.
A huge thank you to our team at Oak and to Owen Henkel (Oxford University), Manolis Mavrikis (UCL), Heather Blicher, Sarah Elizabeth Schwettmann (MIT), and Sarah Hansen (MIT) for their valuable feedback and support with the paper.
Why not give Aila a go yourself, to see how it can support you to create high-quality, personalised lessons for your classroom.